Three crucial prerequisities for data-driven decision making
Over the past five years, digitization has taken off in many supply chain segments, which has opened up both better visibility and data analytics, with a breadth that truly enables a higher degree of qualitative, data-driven decision-making. But often some of the crucial prerequisites for success are absent – and what is missing are not the technical prerequisites.
In the early 2000s, everyone was talking about data-driven supply chains, but usually in terms of Supply Chain Visibility and Supply Chain Analytics. The latter is based on the idea of moving from descriptive (historical), via predictive (forecast) and towards prescriptive analytics. The problem was that the spread of supply chain digitization was very limited at the time, so the analysis was limited or performed on old data from poorly updated business systems.
Hyperautomation
Over the past five years, digitization has really taken off in many supply chain segments, which has opened up both better visibility and data analytics, with a breadth that enables a higher degree of qualitative, data-driven decision-making. In previous articles in SCE, I have written about how valuable the ongoing digitalization trend is in this regard. We now have the opportunity to elevate supply chains from focusing on automation to hyperautomation (an even greater degree of automated decision-making) through access to large amounts of data and the application of AI/ML, as a complement to visibility and data analytics within supply chains as we know them today.
Three critical success factors
Now, however, we have to face new challenges, or prerequisites, if we are to succeed in taking advantage of the fact that the foundations for data-driven decision-making are now starting to come into place. I would like to highlight two prerequisites from McKinsey’s report “The data-driven enterprise of 2025” (published on January 28, 2022 on Mckinsey.com) and another prerequisite based on my own experience from over 20 years in the industry. I consider all three of these points to be critical to successfully reaching a higher degree of data-driven decision-making in supply chains, and if you manage them, you too will succeed. Note that none of the points below involve technical prerequisites.
- Clear ownership. There must be ownership and it must be clear. For example, McKinsey highlights that ownership should lie with the CDO (Chief Data Officer) and this role must be tasked with building value within the company, that it is a role with a clear responsibility for results. Thus, the area also becomes a product (or rather a service) that the company makes money from through the values that the service creates.
- Leave the silo-based mindset. We need to leave the silo-based mindset behind and start sharing data to a very large extent (in another point McKinsey also highlights how they view data security issues and data rights issues, but that’s a whole area in itself and we’ll leave that for another article). McKinsey envisions the formation of platforms for data sharing in industries and other types of member-based spheres of influence, where members’ data is brought together into a whole that further improves the conditions for data-driven decision-making among members. Industries such as the automotive industry, where I have very good insight through my involvement in PipeChain, have the opportunity to live up to this prerequisite.
- Understand your value-drivers. We need to understand our company’s value-drivers – i.e. what ultimately differentiates the company from the competition, makes the company’s products/ services something that customers have to have, the customer service that creates uniquely loyal customers etc. – and seek data sources with data that can inform decisions that make the company’s value-drivers ever stronger. Here I feel that many companies are misguided. They find it difficult to define what data they are looking for, what the analysis model should look like in order for it to capture patterns and draw conclusions that make the company’s value-drivers stronger, neither can they define who within the company actually owns both the issue itself and the process of driving it forward with the help of increasingly better access to data and, not least, relevant data.
Today, it’s obvious to most people that things are starting to fall into place in order to really talk about data-driven decision-making in supply chains. As so often when we in the supply chain business talk about great opportunities for improvement, it is not about a lack of either technology or knowledge. Instead, it is about mainly human issues, including how we create the right conditions for our organisation to move in the direction that will create the great value that a higher degree of data-driven decision-making entails.
Insights
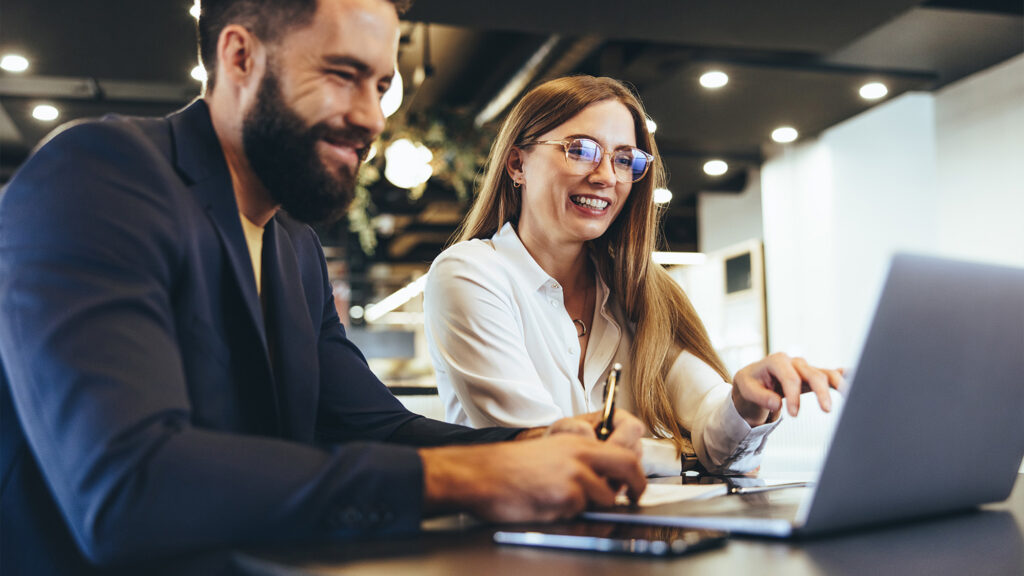
If you are interested in learning more about how to create a powerful connected supply chain, here are our latest insights.
Tech papers
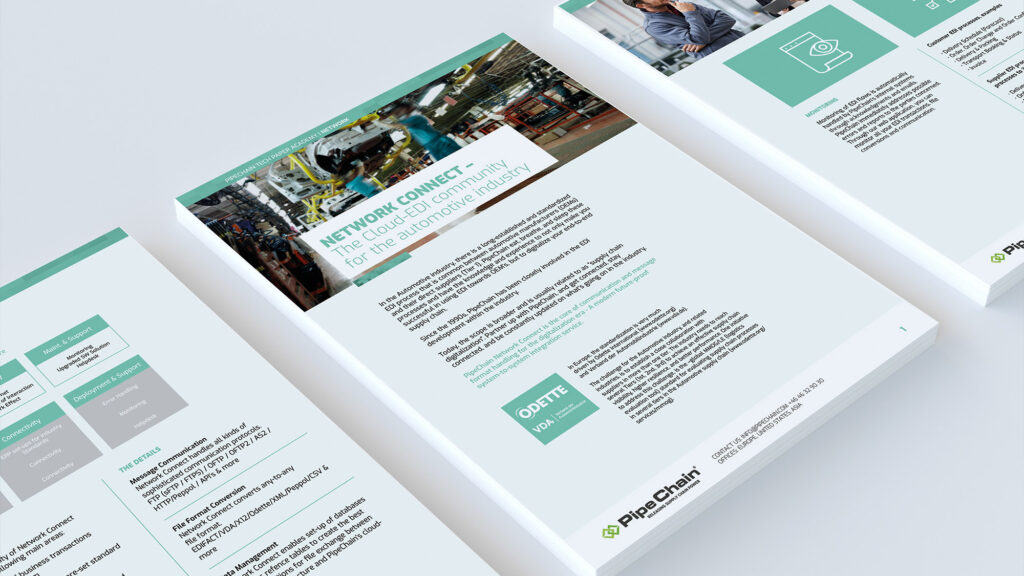
To help you get a deeper and better understanding of our solutions and your supply chain, we have developed a number of detailed tech papers on different subjects.
-
Necessary
These cookies are not optional. They are needed for the website to function. -
Statistics
In order for us to improve the website's functionality and structure, based on how the website is used. -
Experience
In order for our website to perform as well as possible during your visit. If you refuse these cookies, some functionality will disappear from the website. -
Marketing
By sharing your interests and behavior as you visit our site, you increase the chance of seeing personalized content and offers.